First the dog is kicked over, then pushed over, then shoved with a stick. Each time it gets back to its feet.
tv24 desk: But don’t rush to call the animal welfare authorities – it’s a robotic dog undergoing training at Edinburgh University.
Alex Li is the Head of the Advanced Robotics Lab at the university and is among those leading the way in applying artificial intelligence (AI) to robotics.
The AI that controls his dog can cope with situations it has never seen before, like slippery surfaces or stairs.
And if you have ever watched internet footage of robots falling over, then you will appreciate how difficult that is to achieve.
So how did Mr Li and his team train their dog, called Jue-ying, or at least the AI that controls it?
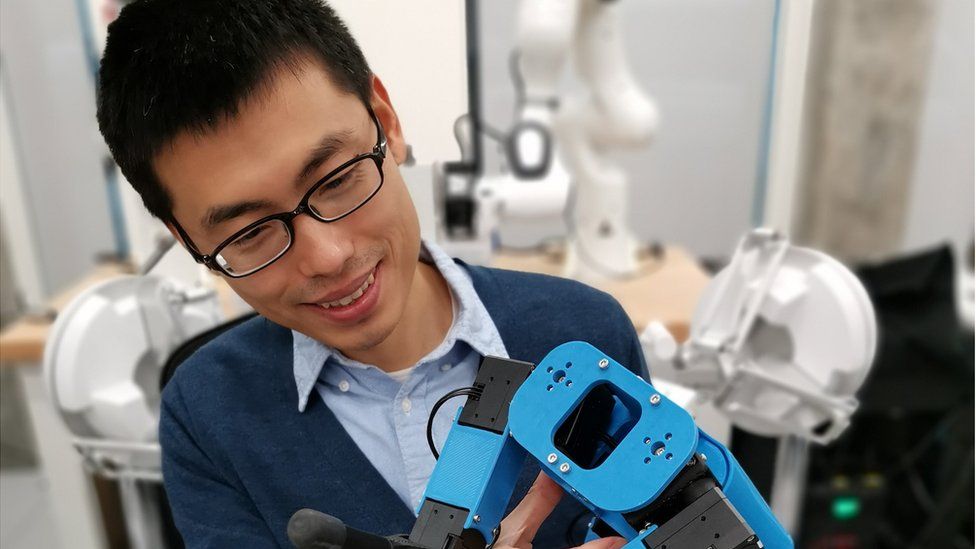
Once they have mastered those basics then they might be let loose in simple matches, where they will learn how to put those skills together to win a game.
That way of learning, which is so natural to humans, is something that companies and researchers are trying to replicate in machines.
The robotic dog was initially taught two skill sets – fall recovery and trotting and walking, and each of those was developed in a different artificial neural network.
Neural networks rely on layers of thousands or millions of tiny connections between nodes, clusters of mathematical computations and can adapt as they are trained.
Those first two skill sets were used as the basis to create others – in total eight neural networks.
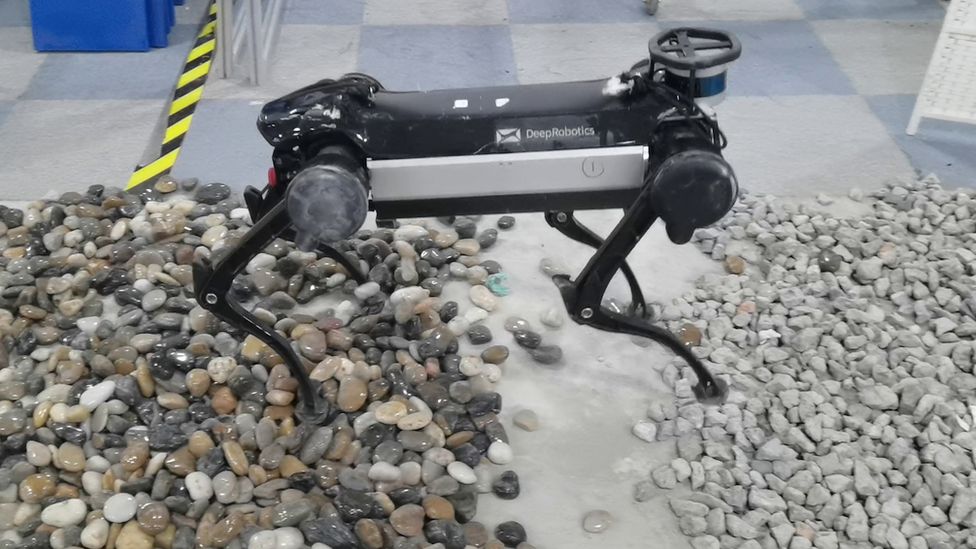
The beauty and potential usefulness of the technique is that the robotic dog could be introduced to completely new scenarios, like navigating stairs or a rocky surface, and could make lightning-quick adjustments to stay upright and continue to its goal.
It might not sound like much but, Mr Li hopes the method can be developed so that robots can complete much more complex tasks.
“Of course locomotion is cool, you can see the robots running around getting kicked in and getting up. But by the end of the day, you want the robot to do something useful for you,” he says.
That will require the addition of features like vision systems and robotic hands, which adds many levels of complexity.
Mr Li’s work builds on research by DeepMind Technologies, an artificial intelligence unit of Alphabet (the owners of Google) and based in London.
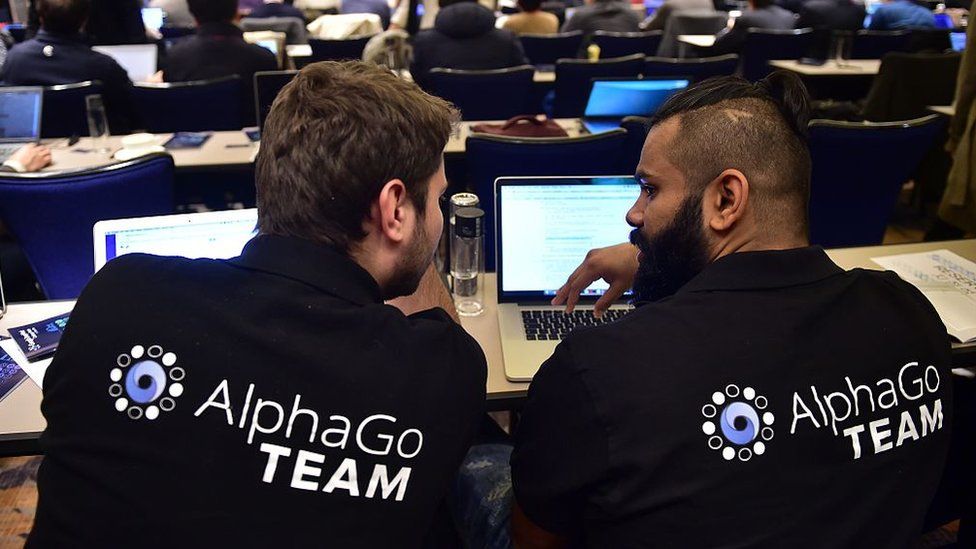
Using that technique, DeepMind has developed AI that has beaten human masters at chess and Go as well as becoming a top player at the computer game Starcraft.
Raia Hadsell is the director of the Robotics Laboratory at DeepMind. She says that combining AI and movement has been a different challenge.
“Your actions change the world,” she points out. So unlike an AI that, for example, plays chess, a robot doing tasks around the home would have to cope with a shifting environment – imagine a robot doing the washing up and using the last of the washing up liquid.
“I think that you will start to see robots being used more with humans in a safe way, because you’ll be able to interact with these robots a little bit more. So they start to be more capable with doing tasks in the home,” she says.
“But probably more significantly, used in parts of industry, agriculture, construction. Imagine being able to enable a farmer with a robot that has general purpose, and could imitate different types of behaviours.”
However, don’t think you can give up ironing just yet.
“I don’t think this is in the next couple of years, but maybe, you know, the next 10 years,” Ms Hadsell says.
Mr Li’s robotic dog senses the world using feedback from its joints and motors – a relatively simple set of inputs. The outputs are just as simple – the dog walks or trots towards a target.
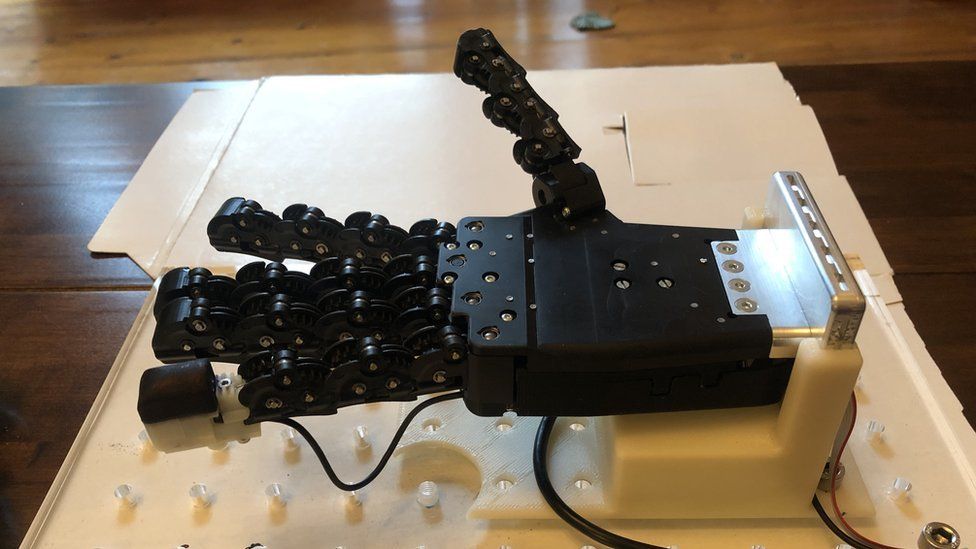
Nathan Lepora is professor of robotics and AI at the Bristol Robotics Laboratory. He has also been training an AI to move, but not a robotic dog, instead a robotic hand that has a sense of touch.
His AI can recognise objects using an artificial sense of touch. While still in its early days he thinks that training AI to sense its environment and move around is potentially very powerful.
“The AI opens up much more general ways of learning how to control rather than, if you like, handcrafting simple controllers. That’s the difference. And that’s what the deep reinforcement learning opens up.
“And deep reinforcement learning also gives the capability to use much more complex sensory inputs as well, for that control.”
However, it’s not going to be easy to train an AI that can control a humanoid robot, equipped with all sorts of different sensors.
“The level of mechanical engineering [involved in] building these robots has kind of gone past our capability to control them, because they’re so complicated. And that’s the problem that’s getting cracked at the moment,” says Prof Lepora. Source BBC